Harnessing AI for Advancements in Nanomaterial Synthesis
Written on
Chapter 1: Introduction to AI in Nanomaterial Synthesis
Researchers from the University of Glasgow have designed an autonomous chemical synthesis robot that explores, discovers, and optimizes nanostructures. This innovative system employs real-time spectral feedback alongside theoretical models and machine learning algorithms to control reaction conditions, facilitating selective templated reactions. By allowing materials to transfer as seeds during exploration cycles, this method broadens the search space in a manner akin to gene transfer in biology.
In an open-ended investigation of seed-mediated multi-step synthesis of gold nanoparticles (AuNPs), the team utilized online UV-Vis characterization, yielding five distinct classes of nanoparticles through approximately 1,000 experiments across three interconnected chemical spaces. This advanced platform enhances nanostructures with targeted optical properties, achieving yield rates of up to 95% by merging experimental data with extinction spectroscopy simulations. Furthermore, synthetic procedures are documented in a standardized format using Chemical Description Language (CDL), which generates unique digital signatures to ensure reproducibility.
The research, titled “An artificial intelligence-enabled chemical synthesis robot for exploration and optimization of nanomaterials,” was published in Science Advances on October 7, 2022.
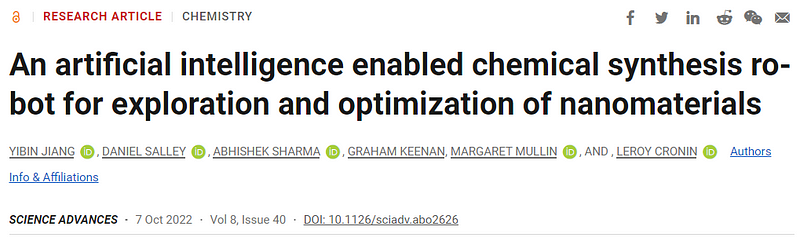
Chapter 2: The Importance of Nanomaterials
Nanomaterials exhibit exceptional physical and chemical characteristics, with tunable size and shape applicable in medicine, electronics, catalysis, and quantum technology. Controlling the morphology of these materials is essential for adjusting their unique properties, including optical, electrical, and magnetic features. Although reliable fabrication methods exist for certain nanoparticles, like gold nanorods, challenges such as non-reproducibility, low yields, and polydispersity persist.
A variety of bottom-up fabrication methods—including electrochemical, photochemical, biotemplate, and seed-mediated synthesis—have been developed to produce nanomaterials with desired traits. However, identifying optimal conditions for specific nanostructures that yield high shape fidelity and monodispersity remains a significant hurdle. This complexity arises from the multitude of synthesis conditions, such as reagent concentration, order of addition, temperature, and mixing rate.
Despite the available synthetic routes, the absence of standardized, robust, and unique digital signatures resulting from the synthesis process exacerbates these challenges, particularly during multi-step synthesis.
The first video, "Artificial Intelligence for Synthetic Organic and Analytical Chemistry," delves into how AI technologies can enhance chemical synthesis processes.
Chapter 3: Advancements in Autonomous Robotic Synthesis
The development of autonomous robotic systems capable of conducting parallel experiments in a closed-loop format, guided by machine learning (ML) algorithms, presents a promising solution to the challenges posed by high dimensionality and sensitivity to synthesis conditions. Recent advancements have led to various autonomous platforms for chemical synthesis, product isolation, and real-time characterization, significantly accelerating material discovery.
However, existing automation in nanomaterial synthesis primarily focuses on user-defined, target-specific optimization and still involves manual interventions. There remains a noticeable gap in systems that facilitate open exploration and the fair search for a diverse array of high-performance products.
Quality diversity (QD) algorithms, such as novelty searches with local competition or multidimensional profiles of phenotypic elites (MAP-Elites), have been effectively utilized in various fields including real-time decision-making and adaptive robotic control. Unlike traditional optimization methods that aim for a single optimal solution, QD algorithms are adept at discovering solutions that exhibit diverse behaviors while maintaining high performance, making them well-suited for exploring chemical space and enhancing product diversity.
A crucial aspect of closed-loop autonomous systems is the selection of appropriate characterization techniques. Various methods, including atomic force microscopy, scanning electron microscopy, and transmission electron microscopy (TEM), are commonly employed to analyze the morphology of nanomaterials. While electron microscopy offers detailed insights into nanostructures, its cost and complexity render it impractical for closed-loop implementation.
The second video, "AI for Chemical Space Navigation and Synthesis - Dr. Connor Coley," explores how AI facilitates the navigation of chemical spaces for efficient synthesis.
Chapter 4: AI-EDISON: The Future of Nanomaterial Discovery
The University of Glasgow's research team conceptualized and built a system for autonomous intelligent exploration, discovery, and optimization of nanomaterials, named AI-EDISON. This system aims to discover and reproducibly synthesize novel nanomaterials across multiple steps, deriving unique digital signatures from both physical properties and synthetic processes.
AI-EDISON's architecture enables the simultaneous synthesis of nanomaterials and real-time spectral characterization, bolstered by machine learning algorithms and an extinction spectroscopy simulation engine. This platform leverages state-of-the-art mass diversity algorithms to navigate the extensive combinatorial synthesis space, enabling open-ended exploration followed by targeted optimization to identify the best synthesis conditions for nanomaterials with precisely tuned optical properties.
By employing a resource-efficient directed graph strategy combined with real-time characterization, AI-EDISON can conduct multi-step synthesis of desired nanoparticles it identifies. This directed graph approach ensures that the comprehensive multi-step nanoparticle synthesis is effectively represented as a robust digital program, minimizing irreproducibility caused by manipulation errors.
The researchers investigated three chemical synthesis spaces linked through seed-mediated synthesis of gold nanoparticles (AuNPs), wherein nanoparticles synthesized from lower-level spaces serve as seeds for higher-level spaces.
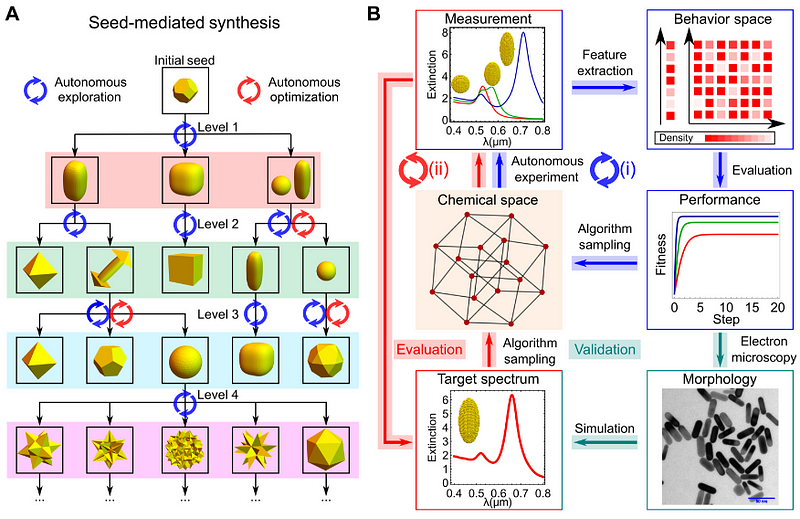
In their exploration phase, the team posited that increasing spectral diversity would facilitate the effective exploration of chemical spaces containing distinct nanostructures. Following this exploration, a simulation engine was utilized to establish targets for further optimization of the optical properties of AuNPs.
The overall algorithmic scheme for nanomaterial discovery encompasses two primary modes: exploration and optimization. In each complete closed loop, AI-EDISON performs three distinct steps that encompass nanoparticle synthesis, UV-Vis characterization, and cleaning, along with designing new experiments using machine learning algorithms.
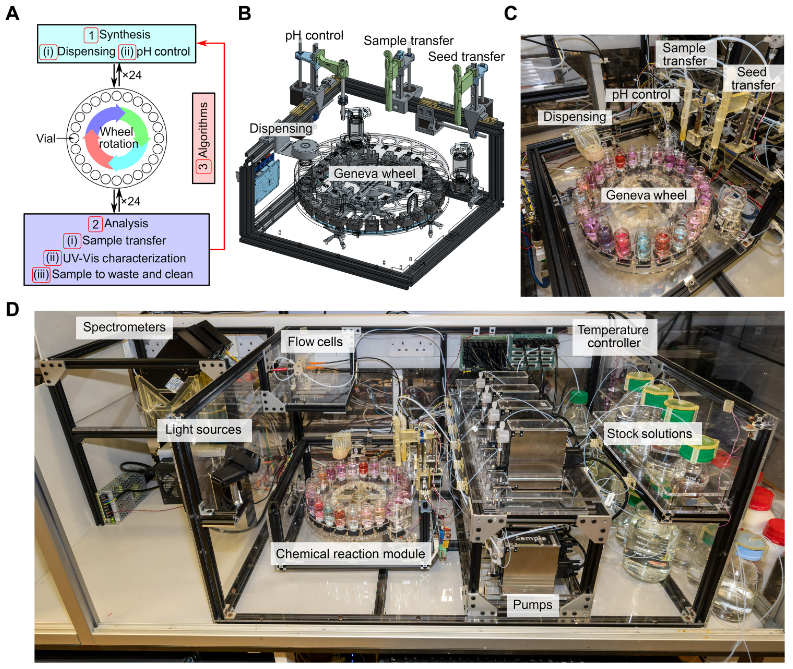
In exploratory mode, the diversity of nanoparticle structures is achieved by seeking variation in behavioral space, which is determined by features in UV-Vis spectra, such as peak count and positioning. The fit serves as a numerical indicator of sample performance, evaluated based on peak prominence and width linked to nanoparticle yield and monodispersity. A new set of experiments is generated from prior synthetic conditions, yielding higher-performing samples with diverse features. This iterative three-step process continues until the exploration phase concludes.
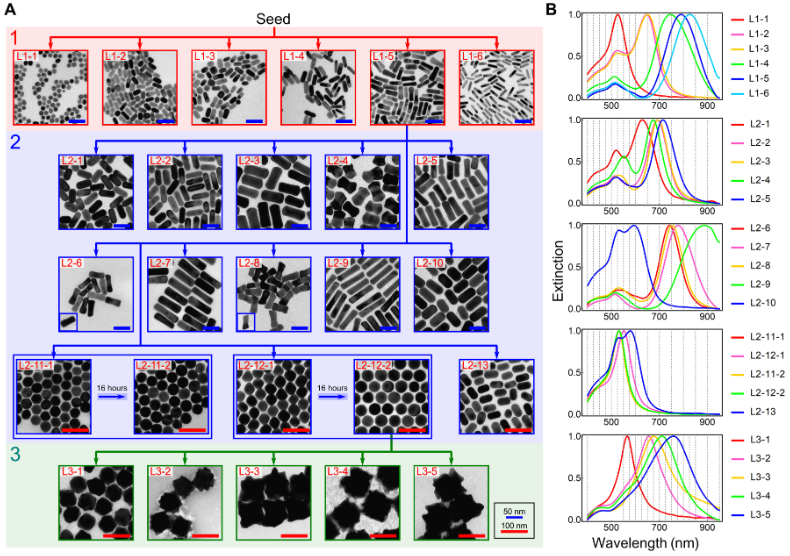
Upon completion of the exploration phase, TEM is employed as a secondary characterization method to analyze the morphology of nanoparticles that exhibit high-performance UV-Vis signatures. In the optimization phase, the target spectrum is defined through simulations of extinction spectra of nanoparticles, with shapes derived from electron micrographs. This strategy expands the optimization goal to nanostructures with features not directly available during exploration.
Given the lack of a one-to-one correlation between UV-Vis spectra and nanostructures, various morphologies may yield spectra that resemble the target spectrum. Consequently, the algorithm accounts for both the similarity to the target spectrum and sampling density within the composite space to identify multiple optimal conditions as solutions to the optimization challenge.
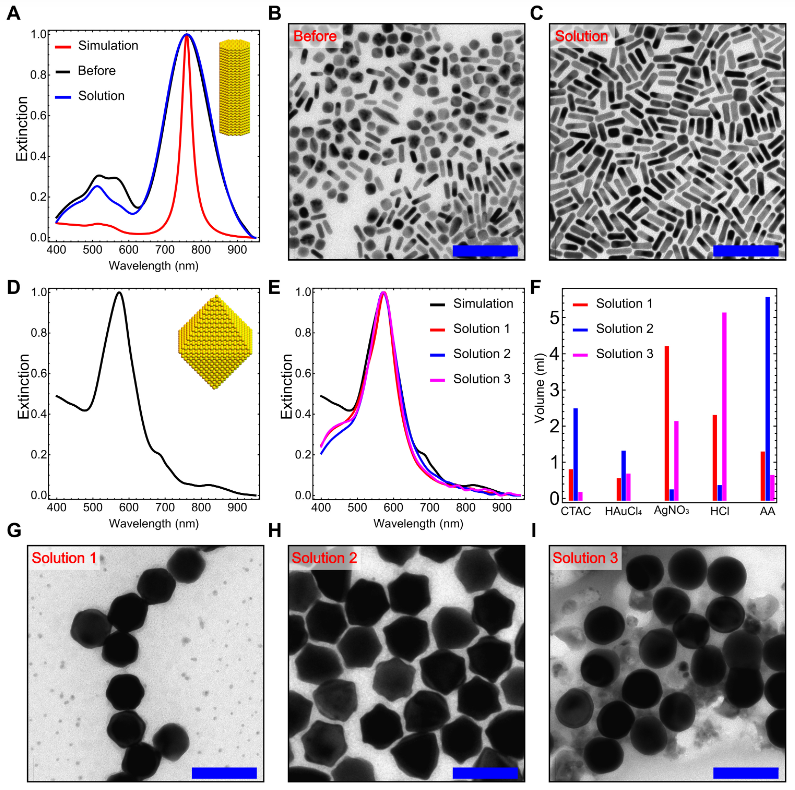